Why Composable and Agile Business Models Need Strategic Business Analytics
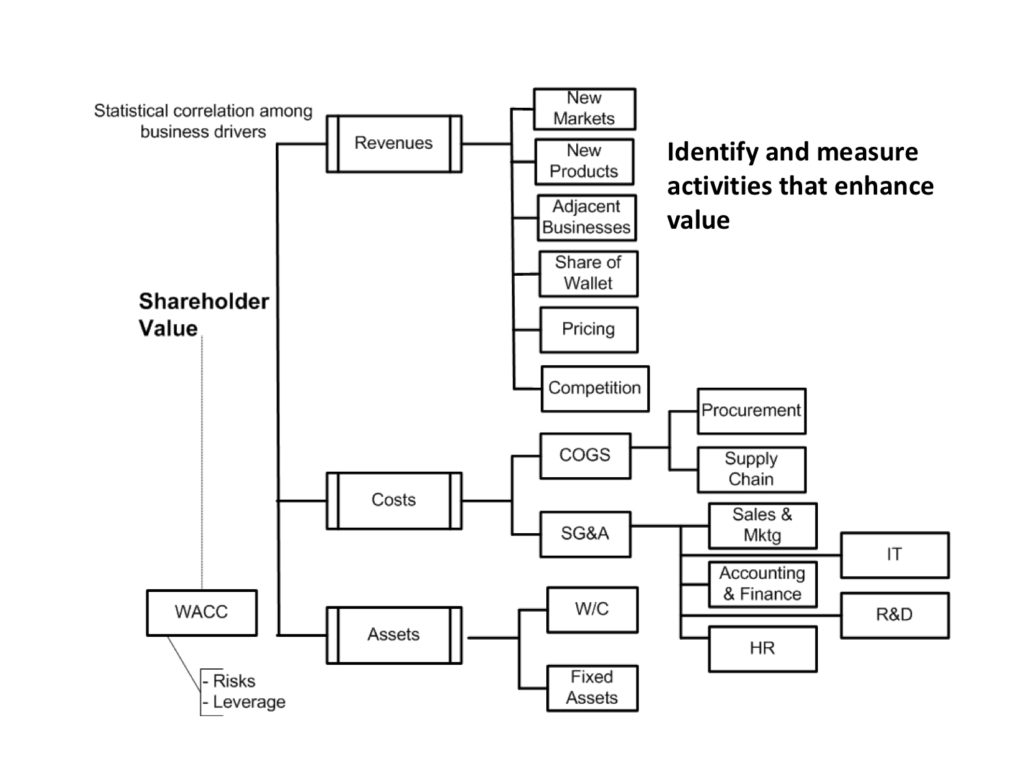
There are two overarching megatrends in today’s market: heightened uncertainty and the explosion of data as we migrate to the digital economy. In these circumstances, employing two crucial tactics in business strategy serves to create business value: 1) composability, the framework and interrelationships among component parts that can be reassembled to transform the business model; and, 2) business process agility, the ability to reconfigure resources and business processes for innovation and scale. Both of these tactics require highly granular visibility into business processes along with analytics to measure performance metrics aligned with business objectives. Given elevated levels of uncertainty around employee health and safety in the age of COVID, and the consequent economic and business uncertainty; a well thought out business strategy analytics framework provides a means of navigating uncertainty with greater confidence and lower risk exposure. To be successful in the face of volatility, the analytics framework employed must be structured to optimize outcomes that drive business value.
Disruption and Uncertainty
The pace of technological change and innovation is accelerating. Exogenous events unfold from numerous factors altering the competitive landscape that radically introduce new business models that in turn, redefine the means of competition. To gain a competitive advantage or even just maintain your advantage, new tools are required. These new tools such as cloud architecture, data engineering, secure data access, scale, analytics and machine learning, are integral in creating the capabilities required to remain competitive.
The rapid transition to the digital economy is only exacerbating uncertainty and the disruption to legacy business models. How can companies prepare and take advantage of new technologies to transform their business? For one, developing a business strategy analytics framework to navigate uncertainty and enhance your business model is a must. Essentially, a business strategy analytics framework is designed to measure performance metrics that are relevant to business objectives while optimizing the outcomes for success. One thing is clear, disruptive innovation and competitive landscape is changing fast, composability, agility and analytics are important.
To offer perspective and context as it pertains to business strategy analytics, the following describes how analytics and machine learning can be applied to address disruption and uncertainty.
First as an example of technological disruption, emerging cellular communication technologies like 5G are set to impact the digital economy – changing the way we do business. Various new business models have already emerged from these new trends, and it is important for business managers to review business model composability and analytics to create new value and new competencies for the digital market.
To assist in this essential process, Green Econometrics professionals focus on distilling the client business model into revenues and costs along with assets and liabilities. So, the task of improving business value is to provide opportunities for revenue upside while lowering operating costs. Processes to improve resource utilization rates and optimize operating efficiencies are critical factors sustaining value. Risk factors also impact business value and reducing uncertainty plays a central role in mitigating risks and improving customer, employee and investor confidence.
It is important to frame any discussion around business analytics and intelligence so that today’s digital divide is thoroughly addressed. The divide facing most organizations now is characterized by the separation of the data side of the equation – encompassing accessibility, integrity, curation, extraction, and transformation of data – from established business logic and operating objectives associated with the client’s business model. Once this separation is addressed, one can develop growth strategies and tactics against which performance metrics are gauged. In this manner, data engineering, analytics and machine learning, and financial analysis are employed as a roadmap to guide the client’s business intelligence strategy.
Digital Economy
The growth in online commerce and digital media are two explosive trends impacting the businesses today. As more companies embrace the digital economy it is imperative to transform data into digital assets and apply business strategy analytics to business objectives. A key inflection point is upon us now. 5G is a nascent wireless communication technology that appears to be quite disruptive to the digital world.
More internet traffic is moving to wireless connections and the tremendous bandwidth advantage that 5G provides will change the way digital services are offered. Apple transformed its entire business model as 3G cellular enabled iTunes and 4G LTE paved the way for extended apps and services for iPhone. The Android and iPhone changed the way people engage with the Internet and as a consequence mobile Internet access is greater than 50% of total Internet traffic volume.
Carriers are beginning to deploy 5G networks and Ultra Wideband services demonstrate significant bandwidth improvements over current connections. Verizon indicated that testing with Qualcomm and Ericsson equipment achieved peak speeds of 5 gigabits per second (Gbps). That’s substantial considering that 4G LTE offers speeds of 10-to-50 Mbps megabits per second, comparable to cable modem speeds in delivery movies and shows. The substantial increase in bandwidth capacity provides the architecture to generate new business models, differentiate service offerings, and reduce transaction costs. When the 5G network infrastructure is deployed, bandwidth capacity could improve by 20-fold. That begs the question: Is there a low risk way to test ideas in developing new business models?
Figure 1 Global Cellular Subscriber
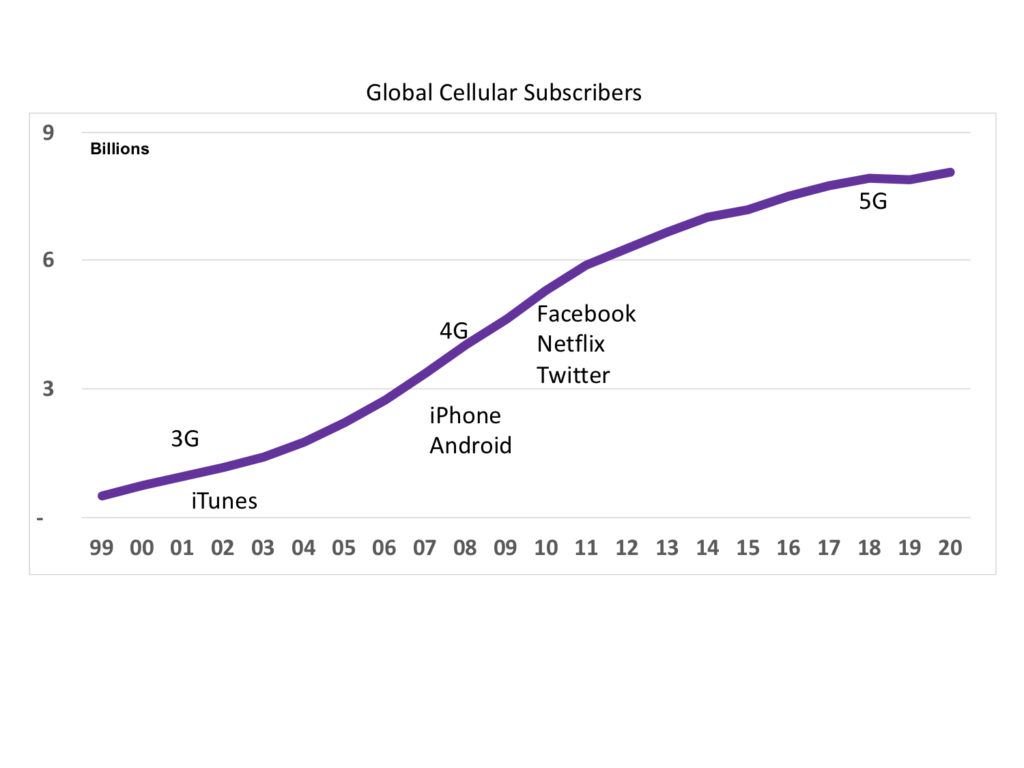
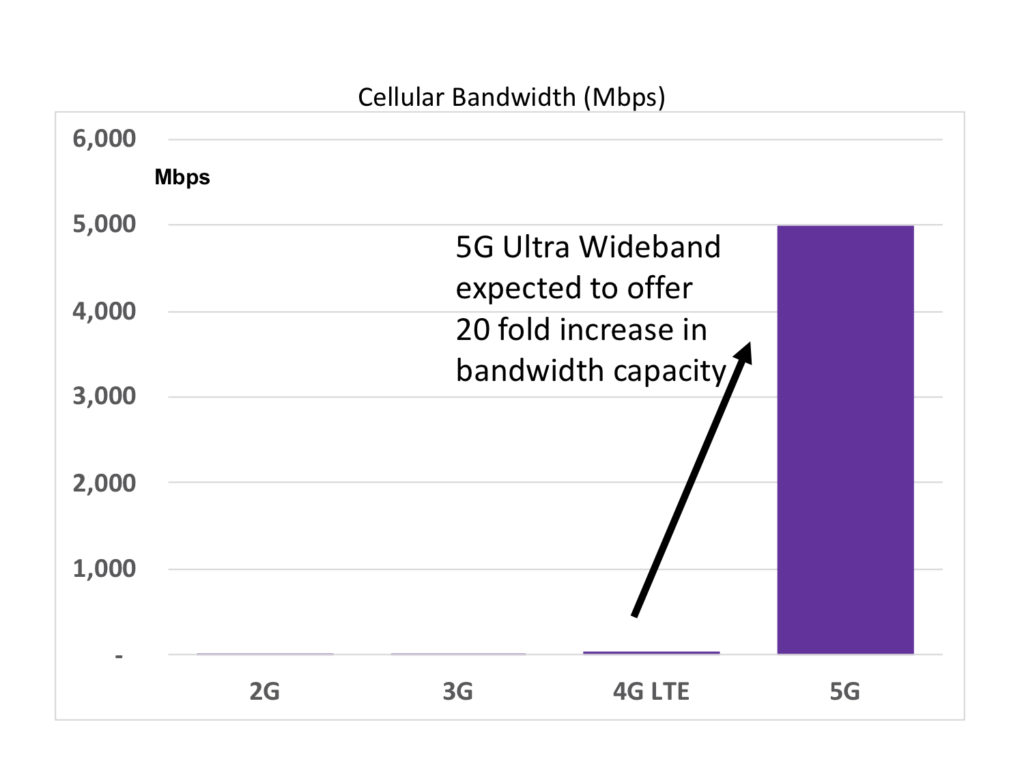
Figure 2 Cellular Bandwidth
With a global population of 8 billion people, there are more cellular subscribers than people. The takeaway is cellular coverage is nearly ubiquitous and as 5G coverage grows, a larger audience becomes available for new mobile service offerings. Therefore, the question to ask is what should you do to prepare your company as the competitive landscape changes and new business models are created? How can you improve competitive intel and become more agile in the execution of your business?
Composability
Composable business models enable transformation of processes and operations to address external threats and introduce innovative new technologies and services to compete in the rapidly changing world. It should be noted that composable business models include financial operations – where revenue sources, costs, equipment and assets can be configured with a focus on factors that contribute to enhancing business value. These factors also include resources, processes, technologies, and strategies. Green Econometrics seeks to build capabilities that produce measurable enhancements to business value by capturing granular data in business operations and processes both internally and externally.
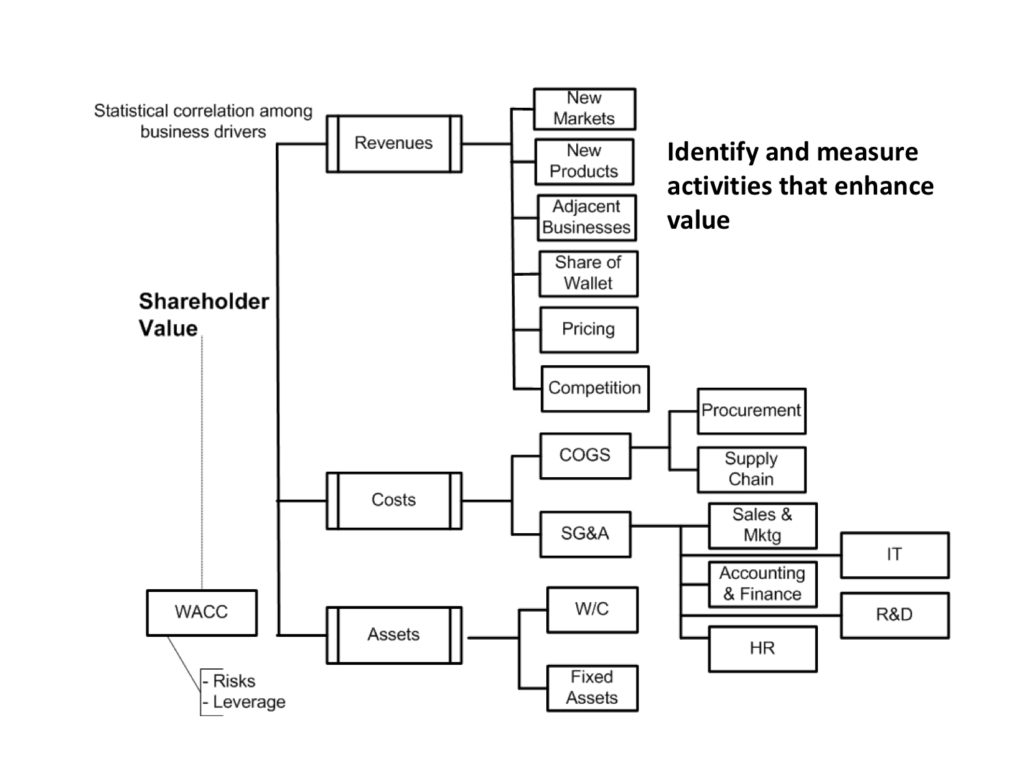
Figure 3 Business Value Drivers
Using data and proxy gauges to assess events, inflection points, and business objectives, an adaptive and more resilient business model can serve to enhance business value. By including inflection points such as leading indicators that could be disruptive to business outcomes as part of the business strategy framework, more dynamic and agile competencies are created. In this fashion, a business becomes more proactive in transforming and reconfiguring resources towards activities and processes that generate value. To effectively reconfigure resources and adapt to changing business models, granular visibility into process and activities across the value chain is required.
There are several technological developments that are driving data analytics and machine learning:
1) The confluence of highly scalable computing and data storage resulting from the availability of cost effective cloud architecture and services,
2) increasing semiconductor processing speeds particularly as they apply to graphic processing units (GPUs) – because GPUs offer the faster mathematical floating point processing required for understanding complex and often non-linear relationships among variables, and
3) The modular availability of sophisticated machine learning algorithms that can be applied to better understand the relationship among variables.
All of the above play a role in reducing the cost and complexity of working with big data. These technological innovations enable more powerful data analytics capabilities and can now be offered as a service supporting secure and encrypted policies.
Today, most machine learning algorithms including structured and unstructured approaches, improve their predictive abilities as the volume of data increases. Machine learning algorithms can be combined into ensembles where predictive power improves, thereby improving confidence and reducing uncertainty. By accessing the right data to create performance metrics, that are relevant to business objectives, one enables composable business models where resources and processes can be reconfigured to enhance business value.
A good starting point in applying an analytics strategy is to start by deconstructing the consumption chain. That is, start with the addressable market for your product or service and work back to suppliers to better measure the performance of each activity and process in your value chain. Clearly this will be different for each organization, but the framework of capturing granular visibility into activities and processes and then applying analytics to optimize outcomes, will serve to improve conditions and reduce risks.
External visibility is also important. One of the best approaches to gauging external visibility is the use of identifying inflection points. The issue with inflection points is that by the time they become apparent, it might be too late to react. Therefore, reducing uncertainty and tying possible outcomes to business impact best relies upon analytics as a resource to more thoroughly prepare for external events and emerging disruptive technologies.
The obvious limitation with inflection points, or any reliance on visibility into the future, is the unknown or unknown unknowns – such as Black Swan events. To reduce uncertainty, proxy measures are helpful in identifying potential event drivers. Again, the point is to reduce the uncertainty of possible outcomes by employing machine learning to help mitigate overall uncertainty.
Business Strategy Analytics
There are several underlying components to building competencies in the digital economy and chief among those components is understanding data: in particular, gaining timely insight to large volumes of data and the varieties of data sources. The business strategy analytics framework is straight forward, and uses data and metrics to guide decisions. Better yet, it optimizes outcomes to align with business objectives
Granular transparency and visibility into operations is a great starting point. After all it is visibility into process that provides the performance metrics required to measure and benchmark value creation or at least contribute to enhancing value activities. Incorporating cross disciple functional activities including finance, operations, marketing, and engineering onto a single pane of glass, i.e. a balanced scorecard dashboard, provides the necessary transparency for the entire organization to understand their contribution to generating business value.
Agility requires instilling a data analytics culture. That is, clearly communicating why we are using data and for what purpose. The broader and deeper the organizational understanding of relationships among various processes in the business and their respective contribution towards goals and objectives, the faster those talents can be reconfigured and applied to address the digital economy and disruptive competitive threats.
Competencies
The business strategy analytics framework together with operating processes, a data analytics culture, and goal-tuned optimization algorithms can engender a significant competitive advantage. These competencies enable product innovation, stronger customer engagements, more efficient operations and better understanding of business value creation.
What is important to note is the substantial role intangible assets play in valuing a business. During a business acquisition goodwill is recognized as the premium paid in the acquisition of intangible assets usually associated with brand name, employee skill, or customer affinity. Intangible assets represent a growing portion of business value as represented by the amount of goodwill in the S&P 500. The trend is illustrating that a growing portion of total assets on corporate balance sheets are categorized as goodwill. This growth in intangible assets reflects the value of intellectual property (IP) and is more important in competitive threats in the digital economy. The development of digital talent, data analytics and integration of business strategy will play a growing role in the digital economy and enhancing business value.
The business strategy analytics framework provides a guide to developing these digital assets and the consequent competitive advantage by identifying potential disruptive events and applying decision support using probabilistic outcomes based on data. These capabilities will expand market opportunities and enable efficient operating procedures to address these markets along with the agility to apply resources based on business objectives and expected outcomes. Learn more about how a data analytics culture can impact your business.
Summary
Developing business strategy analytics that combine composability, the ability to reconfigure resources, and business processes for innovation can help organizations adapt to the uncertainty and complexities of the new digital economy. And provide the agility to adapt and scale along with the competencies that engender employee adaptability and creativity.
A Green Econometrics analytics framework helps businesses navigate uncertainty with greater confidence and lower risk. The key takeaway is that the competencies developed through implementing a data-driven analytics strategy create value in the business, people, process efficiencies and innovation.
Green Econometrics provides research and consulting services regarding data analytics. Green Econometrics’ analytics approach is to identify and define relevant metrics that are aligned to business objectives, measure and benchmark performance, while optimizing outcomes based on machine learning algorithms. We help to benchmark performance with respect to business value creation such as process improvement and operating efficiencies. Part of the business strategy analytics framework may include sustainability metrics regarding environmental conditions such quantifiable ESG metrics including indoor air quality and energy efficiency. Sustainability, operating conditions and performance are all in our wheelhouse.
Related Posts